1D Laplace Equation#
# This is only valid when the package is not installed
import sys
sys.path.append('../../') # two folders up
import DeepINN as dp
import torch
Using default backend: PyTorch
Using Pytorch: 2.0.1+cu117
Geometry#
# A simple 1D geometry
X = dp.spaces.R1('x')
Line = dp.domains.Interval(X, 0, 1)
left_bc = dp.constraint.DirichletBC(geom = Line,
function = lambda X: torch.tensor([0.0]),
sampling_strategy = "grid",
no_points = 1, # you can use more points. there are conditions to deal with stupid conditions.
filter_fn = lambda x: x[:] == 0.0)
right_bc = dp.constraint.DirichletBC(geom = Line,
function = lambda X: torch.tensor([1.0]),
sampling_strategy = "grid",
no_points = 1, # you can use more points. there are conditions to deal with stupid conditions.
filter_fn = lambda x: x[:] == 1.0)
interior_points = dp.constraint.PDE(geom = Line,
sampling_strategy= "grid",
no_points = 20)
# dp.utils.scatter(X, interior_points.sampler_object(), dpi = 50)
# dp.utils.scatter(X, left_bc.sampler_object(), dpi = 50)
# dp.utils.scatter(X, right_bc.sampler_object(), dpi = 50)
PDE#
def laplace(X,y):
"""
1D Laplace equation.
u__x = 0
"""
dy_x = dp.constraint.Jacobian(X, y)(i=0, j=0)
dy_xx = dp.constraint.Jacobian(X, dy_x)(i = 0, j = 0)
return dy_xx
domain = dp.domain.Generic(laplace,
interior_points,
[left_bc, right_bc])
Network#
activation = "tanh"
initialiser = "Xavier normal"
layer_size = [1] + [2] * 1 + [1]
net = dp.nn.FullyConnected(layer_size, activation, initialiser)
model = dp.Model(domain, net)
optimiser = "adam"
lr=0.001
metrics="MSE"
model.compile(optimiser, lr, metrics, device = "cuda")
/home/hell/Desktop/repos/DeepINN/Tutorials/5. FCNN/../../DeepINN/geometry/samplers/grid_samplers.py:78: UserWarning: First iteration did not find any valid grid points, for
the given filter.
Will try again with n = 10 * self.n_points. Or
else use only random points!
warnings.warn("""First iteration did not find any valid grid points, for
Domain compiled
Network compiled
model.optimiser_function, model.lr, model.metric
(torch.optim.adam.Adam, 0.001, MSELoss())
model.train(iterations = 500)
Iteration: 1 BC Loss: 0.0286 PDE Loss: 0.0000 Loss: 0.0286
Iteration: 51 BC Loss: 0.0061 PDE Loss: 0.0000 Loss: 0.0061
Iteration: 101 BC Loss: 0.0021 PDE Loss: 0.0000 Loss: 0.0021
Iteration: 151 BC Loss: 0.0004 PDE Loss: 0.0000 Loss: 0.0004
Iteration: 201 BC Loss: 0.0001 PDE Loss: 0.0000 Loss: 0.0001
Iteration: 251 BC Loss: 0.0000 PDE Loss: 0.0000 Loss: 0.0000
Iteration: 301 BC Loss: 0.0000 PDE Loss: 0.0000 Loss: 0.0000
Iteration: 351 BC Loss: 0.0000 PDE Loss: 0.0000 Loss: 0.0000
Iteration: 401 BC Loss: 0.0000 PDE Loss: 0.0000 Loss: 0.0000
Iteration: 451 BC Loss: 0.0000 PDE Loss: 0.0000 Loss: 0.0000
Iteration: 501 BC Loss: 0.0000 PDE Loss: 0.0000 Loss: 0.0000
Training finished
Time taken: 'trainer' in 3.1389 secs
# model.iter = 1
# model.train(iterations = 2000)
model.network
FullyConnected(
(activation): Tanh()
(linears): ModuleList(
(0): Linear(in_features=1, out_features=2, bias=True)
(1): Linear(in_features=2, out_features=1, bias=True)
)
)
coordinates_list = dp.utils.tensor2numpy([model.collocation_point_sample, model.boundary_point_sample])
solution_list = dp.utils.tensor2numpy([model.collocation_forward, model.BC_forward])
history = model.training_history
import matplotlib.pyplot as plt
plt.figure(1)
plt.scatter(coordinates_list[0], solution_list[0], label = "collocation points", color = "red")
plt.scatter(coordinates_list[1], solution_list[1], label = "boundary points", color = "blue")
plt.grid('minor')
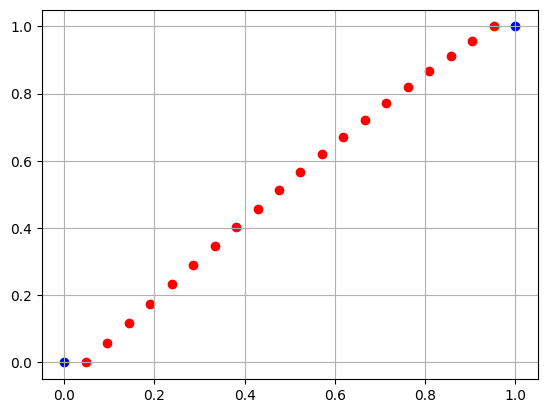
plt.figure(2)
plt.plot(history)
plt.xlabel("Iterations")
plt.ylabel("Loss")
Text(0, 0.5, 'Loss')
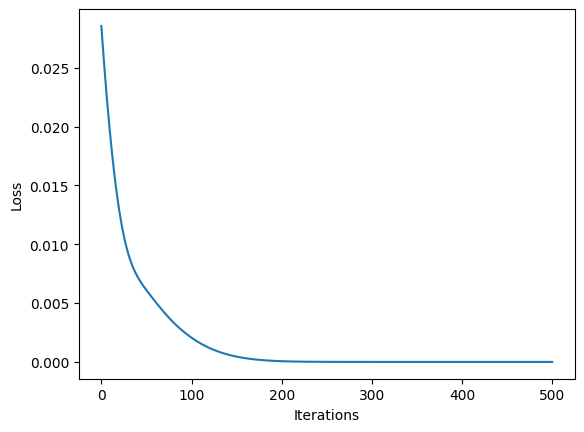